Beyond Stare Decisis: An AI Preparedness Framework For The Judiciary - II
- websitenlsir
- 7 minutes ago
- 17 min read
Hemanth Bharatha Chakravarthy*
PART II
Part I laid the conceptual foundation for judicial AI, first by breaking legal work into retrieval versus inference tasks, and then by introducing a clear three-tier ontology. Through illustrative examples, I demonstrated where AI can enhance efficiency and where human judgment remains indispensable for empathy, context, and normative discretion. Such a framework moves the conversation beyond vague notions of ‘AI in courts’ to concrete categories that clarify both opportunity and constraint. With these insights, this part explores the associated risks AI poses and outlines a strategic, step-by-step agenda for responsible adoption of AI in the judiciary.
An Updated Risk Framework—Moving Beyond “Bias”
Anecdotally and from conversations with hundreds of lawyers, my experience is that the legal studies risk framework for AI technologies lags behind by at least one generation. I have listened to presentations about anonymisation and privacy to remove PII from sensitive data, oblivious to developments in differential privacy and events like the Netflix reconstruction attack. A similar mishap occurs when we limit our maximal imagination of AI risk to bias, particularly regarding machine learning models that process (often high-dimensional) numeric or coded data to predict trends and outcomes. These kinds of models are the easiest to understand and detect bias in. Our autoregressive LLMs whose personalities are artificially reinforced are a substantially different beast. LLM-era models are highly capable but are also heavily incentivised into servility and likability. By making humans rank options in terms of preference in taste, ethics, and personality, we slowly moderate raw models into their saleable personalities.
Various labs and academics have written about alignment and safety. However, as residents of a postcolonial state and emerging market like India, there are other short- to medium-term issues that should preoccupy an updated risk framework. My fundamental worry is that if AI agents become affordable and reliable enough to handle much white-collar work, we may face a worrying “looping” phenomenon. Economically, this could mean that returns to labour are swallowed by returns to capital. That both consumer and service provider are now the wealthy; politically, culturally, and linguistically, it could result in AI standardising new knowledge. In a globalised world, India's economic and cultural growth has vastly benefitted from being the seller to an American or international buyer. But what if the buyer and seller now converge, and various kinds of capital loop within?
Economically, India’s model of upward mobility has substantially relied on services and, particularly, the export of services. A common upward mobility story in India might be growing up in a village, paying to attend a private university, and moving to a city with a low-paying corporate job, perhaps working in BPO or software services. But this move still represents a substantial shift into the middle class, and those who persisted in the system for long enough, did well. If AI outperforms a lot of offshore low-skill white-collar labour, what happens to this upward mobility story? Today, Indian IT is roughly 8% of India’s GDP but also over 52% of the global IT offshoring market, and Goldman Sachs thinks that India has the fastest-growing service exports in the world. Unfortunately, the reigning paradigm in AI is one of scale. We have found recurring success in improving model quality by increasing things correlated with model size, such as the amount of training data, the number of trainable units (parameters) in the model, or the chain of thought test-time compute spent by the model. This is unfortunate because scaling is extremely expensive, leading to a shift where returns are increasingly directed towards capital rather than labour or skill. Both the customer and the model provider reside in California. This is the existential risk in economics from “looping.”
Culturally, the widespread adoption of AI in judicial settings risks producing a uniform legal discourse, potentially flattening a pluralistic Indian jurisprudence into a monolithic and homogenised narrative. An AI system, particularly one trained predominantly on Western jurisprudence or globalised English corpora, risks eroding diversity of thought. Over time, judges and lawyers who rely on these standardised AI-generated opinions and drafts might unconsciously align their reasoning and expression with these outputs, gradually narrowing judicial creativity and interpretative variety. These are the ten- and fifteen-year horizon issues that judiciaries must prepare for today.
Politically, this looping effect extends the subtle influence of private, often foreign, AI companies over domestic public institutions, including the judiciary. When judicial writing styles, terminologies, and even reasoning begin to converge toward AI-generated standards, it inadvertently grants significant cultural power to the designers of these AI models—often private, overseas entities. This indirect influence can be profound, shifting the locus of interpretive authority and subtly reshaping legal norms without explicit public or democratic oversight. The critical insight for action here is to ensure the provenance of the data and personality powering AI pipelines, ensuring that they have the appropriate local context and proactively digitising records to build deeper databases for future systems.
So, as in the creation of any lingua franca or nomenclature, standardisation is a double-edged sword. On one hand, it can democratise access to legal information, codify it to be increasingly machine-readable and usable, and make legal outcomes predictable. It might mean substantially faster justice and a more predictable rule of law. On the other hand, this dynamic tension—between standardisation’s benefits in clarity and interoperability, and its risks in homogenising complex socio-cultural landscapes—makes proactive influence of the judiciary in legal AI efforts essential.
In the case of predictive bias, we noted that old-school algorithms that read lots of numbers about a defendant (or a healthcare recipient) to predict the sentence (or cardiovascular risk) often end up learning models that replicate racial biases or other ascriptive discriminants (see note 5, and Sendhil Mullainathan’s work above). But humans are the sources of these biases and currently display them too, except that it is easier to observe and correct when the algorithm is racist because this can be tested and measured at scale on historic data. This is especially so because predicting a number, category, or True/False is easy to count and scrutinise. These models also have fairly interpretable architectures. Hence, it is possible to benchmark and improve bias rates.
The LLM-era inscrutable analog for bias is the beast of linguistic standardisation. To avoid omission-commission bias, we must again compare the rate at which a problem occurs in computer system against existing human rates. The current system of coded English laws with a top-down systematic enforcement also produces standardisation that is sometimes erosive of local nuances and pushes towards codification and predictability at the cost of diversity. Whereas in the case of predictive bias, humans were difficult to observe and correct, simple AIs could be benchmarked and revised Language models are relative blackboxes; they are not easy to understand or scrutinise. GPTs train by predicting masked tokens and learning which parts of the prior sequence of text they should pay attention to; and in learning to finish complex sentences, they end up learning vast quantities of human knowledge. A GPT’s process encodes texts into high-dimensional numeric spaces, where sequences of neuron activations trace logic patterns that produce maths in that projected space. Much like watching brain activity under MRI, we can only watch prompts take pathways through the model’s layers to find the best completions to prompts. It is not easy to prove what their latent worldviews are, or to deterministically control model ideas and values. Our current approach has been to produce masks of aligned personality via reinforcement learning from human feedback where we punish and reward the model post-training to reinforce good virtue. We attempt to elicit neutrality from these models by masking them past knowledge to truthfulness and alignment.
AI systems will participate in creating the next generation of written language and language imprints memory and ethics. The appropriate field of research to be obsessed with today then is not debiasing, it is alignment research. Mechanistic interpretability[1] wrangles with the subcutaneous influence that our white-collar AI servants will have on us. What produces the personality, opinions, and beliefs of AI systems—who is shoggoth under the mask?
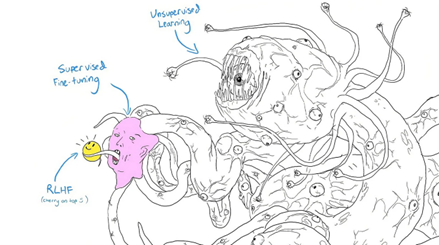
With this updated risk framework, we can ask appropriate questions that help build a preparedness framework for AI in the judiciary. What are the texts and data that the AI systems we use are based in? Does the AI system work on solicitation, or does it work proactively? Does it report speech and summarise neutrally or does it opine? Does the AI diversify its language and writing based on the interaction with the user controlling it? Are there audit trails for AI-generated content that judges read and utilise? These kinds of questions help set a data, procurement, and technical infrastructure agenda for courts to become early influencers in AI etiquette.
The Preparedness Agenda
It seems fair to generalise that humans have historically been able at adopting new technologies. We learn emergent etiquette and language, understand symbols and interfaces, and we make these technologies our own. We repossess them and find our own systems for these technologies. Presumably, this will happen with AI as well.[2]
My argument is that any preparedness framework must be incentive compatible as a prerequisite to be effective. I.e., the institution interested in influencing change and thought leadership must have its own resources dedicated to projects in the space such that it is materially invested in its theories of change. The stakes must be real. This means dedicating administrative efforts towards technical preparation—if we want future systems to be built on rich local data, we need to make various records machine readable proactively. This means expanding efforts to capture and digitise court activity, change-manage e-filing systems, and making judicial data accessible. Legacy data systems like the current NIC architecture are built from the perspective of rigid security rather than fast-moving innovation while preserving integrity. Language models are a combination of the tokens supplied to them—building richer corpora of local legal data means influencing them further towards value alignment. Further, incentive compatibility means dedicating financial resources towards procuring, engaging, and deploying AI products and services at various touch points in the judicial system. If the judiciary wishes for AI products used by lawyers, judges, and registrars to be of good provenance, technically superior, and ethical—they must spend to encourage startup activity in the space. Incentive compatibility also means constructing administrative changes like dedicating AI agendas to high court computer committees and hiring specific decision makers for AI procurement and maintenance in IT and Computers Registries.
Government (as a behemoth) is already the biggest single spender in legal services in India. And it still under-spends. Combined central and state government spending on legal services—including court infrastructure, judges’ salaries, legal aid, and counsel fees—totals roughly ₹25,000–26,000 crore annually (under 0.1% of GDP, with states spending around 0.6% of SGDP), whereas large corporate legal expenditure alone reached ₹63,807 crore in FY 2022–23 and the total size of the Indian legal services market is estimated at around ₹1.2–1.5 lakh crore annually. So roughly, the union and states together finance around one-fifth to one-sixth of legal expenses in the country, perhaps less, giving the state a command over a plurality of legal incomes. This is still low though. Legal aid spend remains critically under‑resourced at just ₹6–7 per capita per year. The AI efficiencies we have been reporting so far can produce substantially larger outcomes at current spend levels and become a powerful public finance intervention.
By early adopting AI systems, the judiciary will both resurrect life in its technical bureaucracy via public-private competition and drive primary private sector innovation via demand. Edler et al. report that government procurement has caused innovation renaissances in European policy tech. Government led efforts like Germany’s with IBM Olga have already found demonstrable success. Innovation also means that the government plays a role as a pooler of risk: with clear mission statements, budget earmarking, and capacity‑building within procurement teams, governments can manage high‑risk, high‑reward contracts. With the right incentives and by allowing bureaucrats forgiveness to experiment and pilot new technologies, mission-oriented procurers can become thought leaders. The Harvard Kennedy School guidebook for result-oriented RFPs reiterates the importance of dedicated administrative talent with flexibility and autonomy to make procurement decisions. Simultaneously governments must invest in change management with various stakeholders across the bar and bench, from registrars to justice seekers, to build behaviors and train technical soft skills needed to use AI effectively and safely (see the McKinsey “revolutionising procurement” report).
So, an effective preparedness framework which produces thought leadership in AI innovation is predicated on participation and early adoption. If institutional procurement is healthy and alive, we can be less worried about risks of relying on third party applications and services for judicial process. Proactive technical teams will be highly capable at ensuring appropriate security and data integrity terms in RFPs and procurement contracts. Ultimately, we cannot afford to be absent when AI becomes the norm and be upset later about the quality of the emergent etiquette. Waiting for technology to become a norm leaves governance lagging behind corporate culture in technical efficiency, except that inefficient governance is a citizen grievance. This is why the OECD has already adopted a framework for government led innovation in AI, the White House has called for judiciaries to adopt AI, and why government efforts to regulate AI are unlikely to succeed without a participatory top-down AI agenda (Zick et al. (2024) highlight more examples of such current efforts like Canada’s CDADM framework and the WEF’s AI Procurement in a Box).
Zick et al. point out that preparedness frameworks on paper often face external validity problems, so it is better to pick good priors and initiate government pilots and procurement. The real world serves as the best laboratory for testing new ideas. Better etiquette will emerge from user culture and users will make a new technology their own. Worries of overreliance on a technology due to the efficiency gains it seems to produce cannot be regulated with firewalls and bans. The internet is ubiquitous, and confidential or sensitive information can easily find its way into personal computers and shadow AI accounts. Preparing others for change also means preparing ourselves for a fresh worldview that is not grounded in luddite nostalgia.
When we prohibit AI systems, we merely make employees their own procurement decision makers. Individual employees will not stop using AI tools, and they will use whatever tools they are advertised to use. I.e., if judiciaries do not procure apt AI systems, their staff will still use AI, but we cannot rely on each individual member to use reliable AI systems of good provenance. On average, it is difficult to know as a layperson whether my queries are being used to train AI models, what privacy settings I agree to, and which tools are fit for the tasks and data that I require. As AI advances rapidly, it should not be the responsibility of individuals to track which AI systems are today’s state-of-the-art. And today or tomorrow, individual judges, judicial officers, registrars, clerks, and interns will use some kind of AI. When procurement is conducted top-down instead, not only do managers get control over the custody and ownership of their data, but they also benefit from their juniors’ AI usage becoming transparent and observable. Most of all, incentives and exposure align such that they can procure AI that is good for stylised tasks, has the appropriate interfaces, and is technically state-of-the-art.
Adept AI procurement requires thinking about which tasks or settings require stylised vertical AI tools and which are performed by general tools. My definition of vertical AI is tools that go past frontier models to provide rich dashboards or interfaces, are powered by up-to-date data coverage (eg., indexing case law and statute), and can independently perform agentic end-to-end legal tasks. Then the first requirement from a potential vertical AI vendor is rich, interactive interfaces fit for the task. For instance, a drafting AI must edit my word document. A document review AI must make comments on my PDF. A litigation tracking system must give me interactive cause lists and docket dashboards. This is true of all vertical AI—a financial agent should output DCFs and charts, a shopping agent should output Amazon orders, and so on. Any workflow that has stylised legal tasks will benefit from an AI that has AI collaborating with humans in appropriate interfaces, mapping various digital worksites to be AI interoperable. Whereas internal administrative flows that have diverse tasks that are not very stylised might benefit instead from broadly capable generalist AIs.
Enterprise AI procurement as a strategy will also become increasingly forgiving on the purse, besides already producing public finance efficiencies. According to Sam Altman, compute costs follow a convex Moore’s Law. If the number of transistors in a chip roughly doubles every two years, AI costs roughly fall about 10x every year. While it will eventually become easier for individuals to access their own hyper-personal assistants, in the early days the state must exploit its pooled bargaining power and acquire technology on behalf of its beneficiaries. When judiciaries assume the cost of compute for access technologies, like AI-generated headnotes or translations in their digital reporters, they are relieving young lawyers, small practitioners, and average citizens from bearing the cost of compute. Thus, enterprise judicial AI adoption becomes a market-making tool that democratises entry costs for new lawyers or firms and makes the legal services industry more competitive.
So, early adoption means judicial officers and staff use better tools provided by managers, while also exposing their AI usage to managerial scrutiny. It means that the government drives innovation and thought leadership, so as to influence etiquettes. A combination of administrative, technical, and behavioral changes will produce a more efficient judiciary while aligning AI innovation. If we agree so far that we should early-adopt enterprise AI, the immediate etiquette to learn becomes procurement etiquette for AI-era technologies. In all the procurement literature cited thus far, the seeded trivial assumption is that we already know from experience that cost-based assessments do not work very well. This is true for AI as it is for building roads and bridges—tenders must balance quality metrics with cost bargains. Especially in AI, where scale is proportional to quality, increase in cost often tautologically means richer outputs. Being an early adopter requires boldness in pursuing high-risk, high-reward procurement as new hardware drives costs down. It means treating technological interventions, especially knowledge technologies like AI, as investments. This does not mean that we must become over reliant on overseas model providers that dictate costs. Developments like Deepseek highlight the effort to find efficiency frontiers that reduce costs for existing scale-based model architectures. India has not yet, but will hopefully soon, emerge as a player in the race for frontier models. Until it does, we must at least invest in vertical AI and application layer systems that are built for Indian systems.
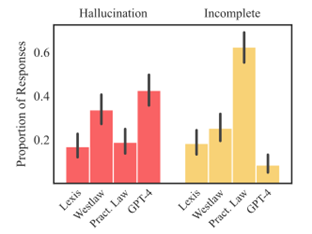
Procurement failures can also arise from more insidious assessment criteria. For example, tenders often slap a minimum number of years or revenue requirements that providers must satisfy. This keeps software providers of the state isolated in a shortlist of legacy services companies. Whereas spiritually, the AI world most canonically represents the complete cultural triumph of the West Coast. The AI world is abnormally driven by hyperscaled startup labs, and more broadly, by industry. Many of its seeds come from nontraditional subcultures. Chris Olah, one of my favourite AI researchers, does not have an undergraduate degree. Top-class PhDs line up at startups and undergrads leave college to join frontier research labs. So, high-risk, high-reward procurement means finding ways to breed stability while working with nascent technologies but also nascent providers.
Obviously, stability is important. Working with a startup represents an existential risk for any government program. This means that procurement decision-makers need to find creative ways to inject stability into their partners. This could come from owning the compute, licensing models, and having dead man’s trigger clauses that leave ownership over data and systems with the procurer if the partner experiences insolvency or liquidation.
Given such relaxed minimum guarantees, procurement agendas should instead focus on benchmarks and performance. Even for debiasing models and making them safer or better aligned, high-quality benchmarks are essential tests. For instance, paralegal AI models must have to take MCQ tests and produce swathes of writing and reading comprehension exercises that are judged by human or algorithmic judges. Unfortunately, our nascent legal tech ecosystem does not yet have good benchmarks or evidence-based studies to evaluate legal AI providers. In the absence of high-quality numeric indicators, it is important for procurement decision-makers to not fall prey to unsubstantiated claims or returns. If a tool does not cite a publicly available study but makes claims about changes in turn-around-times (TATs) or return on investment (RoI), this should account as a red flag. The important thing to remember is that AI system benchmarks must be used to compare providers against each other, but also to compare best-in-class AI systems against corresponding human performance. Benchmarking or studying human performance and pain points will allow courts to identify where there is lots of value to unlock from technology.
In the absence of high-quality benchmarks, it is important to find appropriate proxies. While market presence is a decent proxy, in my experience, this is easily hijacked by marketing capital or players with existing networks. AI is an early, emergent, and powerful technology. It is also built upon blackboxes: in predicting the next tokens (subwords) of a sequence (sentence) across vast training runs scanning through the entire written internet, models somehow develop knowledge and reasoning. Somehow, without understanding neurocognition very well, we have still built a simple simulacrum of the human mind. We are still learning what the failure modes in current models are and how to avoid them: be they issues of snowballing context or missing training data that causes hallucinations or be they issues of personality, agreeability, and censorship. Excellent AI systems are typically built by scientific teams collaborating with jurists to wrangle with large quantities of legal data—AI as a nascent technology cannot yet be reliably built by purely legal (ie., non-technical) teams nor legacy IT companies.
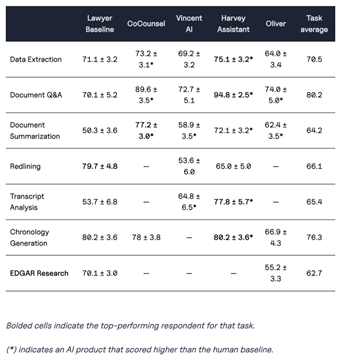
More appropriate proxies might be the development team of a model, its technical specification, and whether it has been substantially beta-tested. Nonetheless, procurement decisions must go through both their own qualitative testing with human expert audiences as well as through pilots that trial and improve AI systems in real-world conditions. To gauge real-world viability, decision-makers must ask pointed questions: Who built the model? What are their qualifications and track record? Is the model retrieving comprehensive and updated data for the legal domain, and has it been subject to transparent beta testing or peer review?
At the same time, AI procurement should never hinge on a single demonstration or marketing pitch. Substantial user pilots are necessary to test how the tool interacts with actual judicial workflows. High courts should share pilot results with each other, and interventions that have succeeded in some jurisdictions should be replicated in others. A generative AI system might perform impressively in a canned demo but could struggle when faced with domain-specific intricacies or large volumes of unstructured documents. Human-in-the-loop testing—where judges, clerks, and legal officers simulate real tasks and provide feedback—is essential to ensure the tool not only functions well in theory but also integrates smoothly into day-to-day judicial operations. If the model’s performance degrades over time or fails to align with institutional ethical standards, procurement contracts should allow for re-evaluation or termination without undue burdens. We need to change our procurement lens and methods to be one that enables flexibility and change.
Conclusion
Judiciaries, but also other social contracts of the nation state, must be rethought for the AI era. We must decide what tasks must be reserved for human self-determination, and where we must enshrine our human right to make mistakes and ability to intuit situations. Our motivation for what makes the human psyche unique could be whatever it is, be it a non-materialist interpretation of consciousness or a belief in the unique, subversive, and empathetic nature of emotional and somatic experience. But as long as we can agree on what tasks we do not want to outsource to thinking machines, we can stay clear of major controversy. Such tasks might be those involving a contextual understanding of socioeconomic circumstances and an application of emotional connection like in the case of awarding sentences or compensation. But there is a broader ontology of judicial tasks and AI is really fit for purpose for so much other legal process. So, if the judiciary decides to embrace data interventions, embrace AI through enterprise procurement, and makes wise decisions to pick technically apt solutions … we might just get lucky.
*Hemanth Bharatha Chakravarthy is the Co-Founder & CEO of jhana.ai, which produces datasets, agents & interfaces that make legal research, drafting & doc review faster. He trained in applied mathematics and economics at Harvard College and has a background in quantitative research, policy, and government.
[1] See for example, the Transformer Circuits Pub exploring what makes transformers the intelligences they are by Anthropic: https://transformer-circuits.pub
[2] For example, take the case of political deepfakes. These have been used adversarially, for example, by Erdogan during elections to link opponents to insurgent outfits. These have also been used by mainstream politicians to campaign in absentia, for example, Imran Khan campaigning for the PTI whilst imprisoned via deepfakes. However, in due time, humans will develop a taste for AI-generated content. In not too long, children might look at advertisement banners and call them slop. We will know to look for fingers and limbs and lighting and absurd background elements to detect AI art. Newer models will grow more realistic and accurate, and we too will evolve our sense and taste for human authorship.
Comments